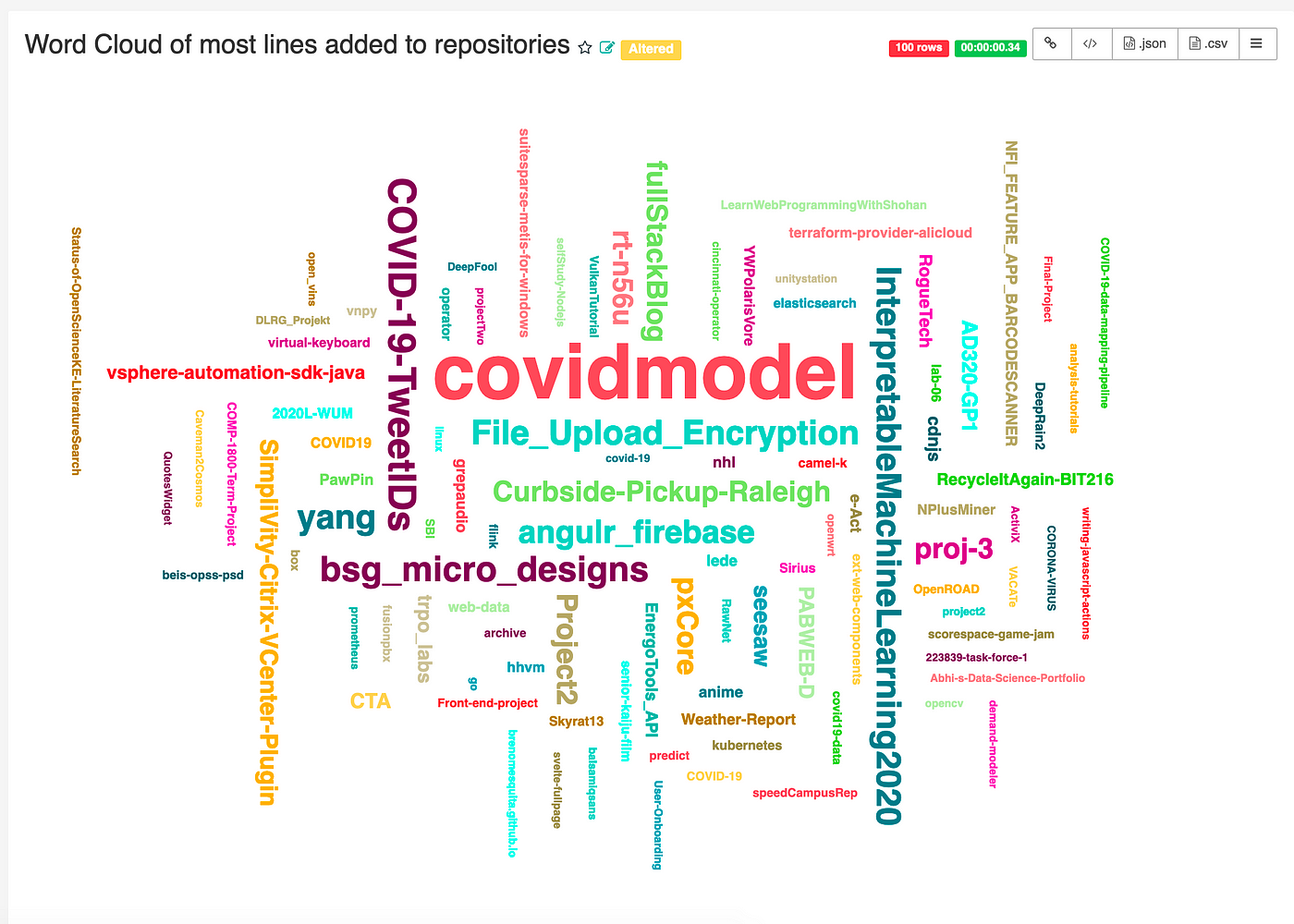
Pinot is the latest Apache incubated project to follow in the footsteps of other tremendously popular open source projects that were first built by engineers at LinkedIn. Pinot joins the likes of Kafka, Helix, and Samza — the former of which is quickly becoming the industry’s message broker of choice for building highly-scalable cloud-native applications.
Outside of LinkedIn, Uber was one of the early adopters of Pinot, using it to power analytics for a variety of use cases such as UberEats Restaurant Manager.
Getting Started with Apache Pinot
In this blog post, we’ll show you how Pinot and Kafka can be used together to ingest, query, and visualize event streams sourced from the public GitHub API. For the step-by-step instructions, please visit our documentation, which will guide you through the specifics of running this example in your development environment.
Pinot Overview
First, let’s do a quick overview of the Pinot components that we’ll be using in this tutorial.
Pinot System Architecture Diagram
Physical components
Zookeeper
Pinot uses Apache Zookeeper to store cluster state and metadata. It is the very first component that needs to come up when creating a Pinot cluster.
Controllers
Controllers maintain the global metadata of the system, with the help of Zookeeper. They manage all other components of the cluster and are responsible for initializing the real-time consumption. Controllers have admin endpoints for managing configs and cluster operations.
Brokers
Brokers handle Pinot queries by forwarding them to the right servers, merging the received results, and sending them back to the client.
Servers
Servers host data segments and serve queries off the hosted data. When the data source is a real-time stream, servers directly ingest from the stream, periodically converting the in-memory ingested data into segments and writing them into the segment store.
Tutorial Overview
Now that you know the basics of Pinot and its architecture, let’s dive into what we’ll be building in this tutorial. Before we review how to ingest GitHub events from Kafka, let’s get familiar with the components we will use to query that data in Pinot. Similar to many NoSQL databases, Pinot has a browser-based query console and REST API. We call this component, the Pinot Controller, and it is the easiest way to run queries outside of a terminal or custom application.
Pinot Controller Start Page
As a part of the Pinot Controller, we provide a query console called the Pinot Data Explorer. If you’re new to Pinot, we have put together a custom docker image and instructions that will help you get up and running as fast as possible.
Pinot Data Explorer
Now that you have a local Pinot cluster up and running, and are able to access the Pinot Data Explorer console. Let’s go over how to create a schema and table that maps a Kafka topic to a queryable data structure in Pinot.
Ingesting GitHub Events with Apache Kafka
Pinot has a variety of ways to ingest data collected from event streams. Today, we’ll be using Apache Kafka to collect event data from GitHub’s public REST API. We chose GitHub events because it is publicly available, there will be a constant stream of events, and it would give us interesting relatable insights about open source projects. We’ll then use Pinot to easily run analytical queries on the aggregate data model that resulted from event streams stored in Kafka topics.
We will be using the /events API from GitHub. In order to get all events related to commits being merged , we are going to collect events of type “PullRequestEvent” which have action == closed and merged == true. For every pull request event that we receive, we will make additional calls to fetch the commits, comments and review comments on the pull request. The URLs to make these calls are available in the payload of the pull request event.
Using the above four payloads, we finally generate a schema for Pinot, with dimensions, metrics and time column, as follows:
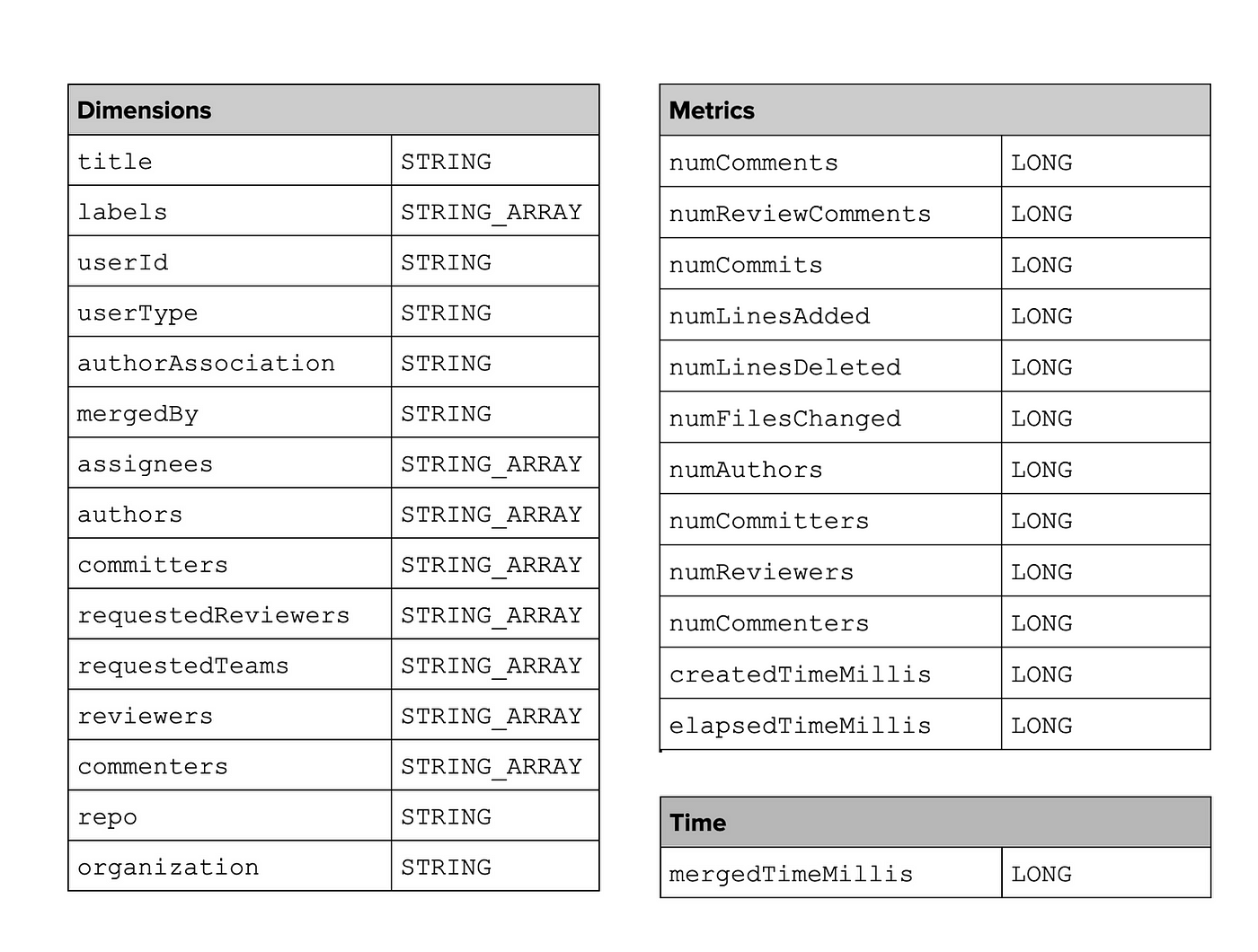
GitHub Event Schema for Apache Pinot
Querying GitHub Events with Apache Pinot
Now that we have our schema and table created, Pinot is able to ingest GitHub events from Kafka so that we can query it as a data structure using PQL. Earlier we reviewed the easiest way to query Pinot from a web browser, using the Pinot Data Explorer. Pinot has a SQL-based query language called PQL (Pinot Query Language), which is a declarative query language that gives you a familiar way to interface with data. It’s important to mention that PQL is based on SQL, and shares much of its semantics, but is not intended to be used for database transactions or writes.
After firing up the Pinot Data Explorer, you’ll be able to run a PQL query to fetch data from the GitHub events that are being ingested in realtime.
Query GitHub Event Schema in Pinot Data Explorer
From here, there are many different ways to interface and visualize the real-time event data from GitHub. One such way is to create a chart using Apache Superset, which is a popular open source web-based business intelligence tool. This is a common tool used to create reports that visualize Pinot data queried with PQL.
Querying GitHub Event Data from Pinot in Superset
You can find more details and instructions on using Superset with Pinot from this community blog post.
Conclusion
In this tutorial we introduced you to using Kafka and Pinot to analyze, query, and visualize event streams ingested from GitHub. Please visit our documentation for the comprehensive step-by-step tutorial referenced in this blog post.
If you’re interested in learning more about Pinot, become a member of our open source community by joining our Slack channel and subscribing to our mailing list.
We’re excited to see how developers and companies are using Apache Pinot to build highly-scalable analytical queries on real-time event data. Feel free to ping us on Twitter or Slack with your stories and feedback.
Slack: https://communityinviter.com/apps/apache-pinot/apache-pinot
Meetup: https://www.meetup.com/apache-pinot/
Twitter: @apachepinot https://twitter.com/ApachePinot
Apache mailing list: dev-subscribe@pinot.apache.org
Finally, here is a list of resources that you might find useful as you start your journey with Apache Pinot.
Docs: http://docs.pinot.apache.org
Download: http://pinot.apache.org/download
Getting Started: https://docs.pinot.apache.org/getting-started
Special thanks
A very special thanks to Neha Pawar and the Apache Pinot engineering team for co-authoring this blog post. If you’re interested in co-authoring or contributing an article to our developer blog, please reach out to @kennybastani on Twitter.